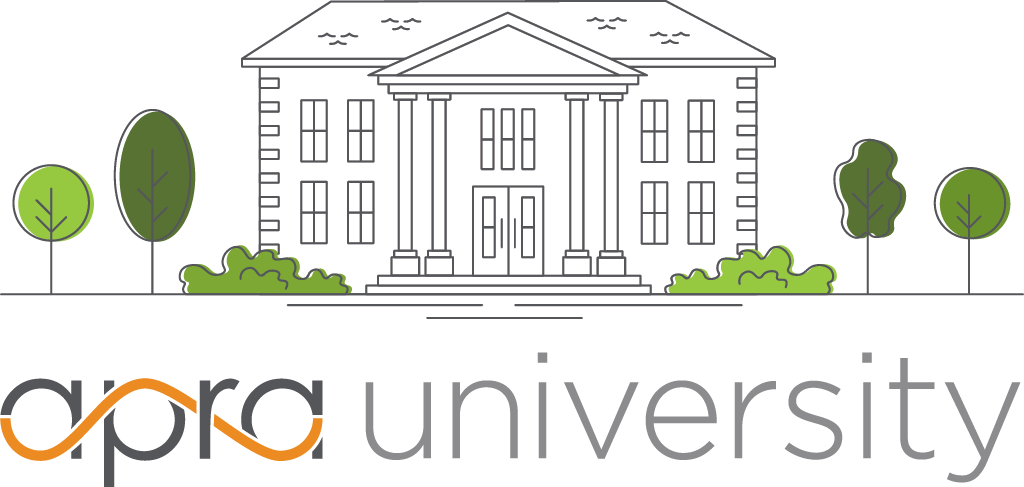
2020 Data Science Now Recordings Bundle
-
Register
- Non-member - $310
- Professional Member - Free!
- Associate Member - $220
Get 10 educational recordings including peer case studies that address all aspects of successful data science integration in development offices, including best practices, project design, resource utilization, and cutting edge applications. Sessions include:
- Fits Just Right: Creating an Analytics Program That is Right-Sized to Your Needs, Talent and Data
- Case Studies: X Never Marks the Spot/Feedback
- Pandemic Perspective: Early Indications and Long-Term Implications for the Data Science Profession
- Building Revenue Forecasts When Your Assumptions No Longer Apply
- Case Studies: Roadmap to Enhanced RFM/Amazon SageMaker
- Data Science Now Panel
- Excel to R: Upgrade Your Skills and Improve Your Workflow<
- Case Studies: NLP/Text Mining in Excel
- Utilizing Data Analytics to Drive Campaign Planning & Beyond
- Case Studies: Mapping in Power BI/Randomistas Deconstructed
-
Contains 1 Component(s)
A reality for many organizations is pursuit of analytics-driven by new data, technology or technical skills. This often results in creating something interesting, but not very impactful: it was created from excitement to innovate and not always aligned with organizational needs. In this session, we will explore an assessment approach that includes reviewing data structures and existing reports, stakeholder perceptions and needs and leadership vision that will help you develop and implement an analytics road map that’s right for your organization. Once true needs and capabilities are determined and prioritized, a road map to impactful and scalable analytics development can emerge and drive a thoughtful culture of adoption.
-
Contains 1 Component(s) Recorded On: 10/06/2020
'X’ Never, Ever Marks the Spot: The Evolution of a Data Science Project James Rogol, Assistant Director of Advancement Business Intelligence, University of Virginia In many ways, the use of Machine Learning and AI are like quests for mythical riches. Commonly romanticized to depict a series of basic tasks promising knowledge or fortunes beyond one’s wildest aspirations, Data Science projects are rarely (if ever) straightforward endeavours. To quote a certain fictional archaeologist, “We do not follow maps to buried treasure.” Previous work, shifting business priorities and technical limitations will inevitably transform the questions and goals underlying a data-driven investigation. More importantly, navigating such different challenges requires unique Data Science skillsets. Rooted in experiences diving into the University of Virginia’s data, learn how the interplay between data analysis, engineering, and statistical rigor can help Data Science projects succeed. The Importance of Feedback in Data Science Natalie Spring, Director, Prospect Research, Management, & Analytics, Duke University This session is a case study that demonstrates how creating an implementation strategy and iterating analytic tools creates stronger client-focused products. Natalie discusses methods of feedback, communication, and stages in implementation.
-
Contains 1 Component(s) Recorded On: 10/06/2020
We are living in a moment of unique opportunity. As we confront uncertainty in the fundraising environment and wade through shifts in expectations, we are also uniquely positioned to weigh critical strategic investments in our future. During the first half of this session, Blackbaud will share key trends that are emerging from organizational and constituent activity data. Attendees will gain perspective on what behavioral changes appear to be taking place and how they can create opportunities to engage with new prospects. Blackbaud will also share a framework for how to interact with your organization's leadership to talk about these trends, create action, and demonstrate your value. Attendees will then have the chance to break out into smaller discussions to talk further about the trends shared and personalize the framework for their own use.
-
Contains 1 Component(s) Recorded On: 10/06/2020
The Decision Science team at Memorial Sloan Kettering Cancer Center has been forecasting annual revenue relatively successfully for many years. But the usual methods rely on the assumption that historical results are likely to be predictive of this year’s outcome for a large part of the operation. This year that assumption does not hold. Kate will describe MSK’s standard approach to revenue forecasting, and how they adjusted their approach for 2020 and 2021, leaving time for a conversation with attendees to share thoughts on revenue forecasting in this environment. Be warned, it’s going to get messy.
-
Contains 1 Component(s) Recorded On: 10/06/2020
A Roadmap to Enhanced RFM Scores: What You Can Do Beyond the Basics (With R and a Little SQL) Andrew McMahon, Assistant Director, Prospect Analytics, US Holocaust Memorial Museum Basic RFM analysis can go along way towards highlighting your best prospects for retention and upgrades. But, two enhancements can make your ratings more timely, granular, and useful to your fundraisers. This case study will introduce these two enhancements and serve as be a roadmap to help you implement them. First, we will cover how to create dynamic scores that update daily in your CRM–This may be easier than you think especially if you have the support of your database team or know a little SQL. Second, we will cover ways to use more involved techniques–like Markov Chains and clustering algorithms–to achieve more granular donor segments; we'll suggest specific R packages to help you implement these techniques. The goal of the case study is to provide an overview of two practical techniques to enhance your prospecting metrics. Predictive Modeling in Amazon SageMaker Maxwell Dakin, Development Analytics, John F. Kennedy Center for the Performing Arts In an effort to cut costs on direct marketing, we used Amazon SageMaker – a cloud-based ML platform from Amazon Web Services – to develop a set of Machine Learning models for prospect identification. We are testing the results in direct mail and email campaigns over the next year, with the goal of reducing costs by bringing model development in-house and focusing our direct marketing efforts on the best leads. In this session, we will cover what we have learned from the project, why we chose this tool and how we plan to implement the results.
-
Contains 1 Component(s) Recorded On: 10/07/2020
Putting the “now” in Data Science Now, this discussion will focus on the current environment prospect development professionals are facing. Panelists will explore the fundraising outlook, covering topics on impactful and actionable diversity and inclusion initiatives, staffing and hiring challenges, and being asked to do more with decreased resources.
-
Contains 1 Component(s) Recorded On: 10/07/2020
Have you ever deleted a column of data that you were sure you didn't need only to realize it was critical? Have you ever struggled to remember how you came up with that prospect pool? Have gift officers ever questioned why a prospect ended up in their portfolios? Microsoft Excel is a powerful, industry-standard tool, but is it the best tool? R, as a programming language, can appear daunting, but this open-source software can provide incredible benefits for those willing to take the plunge. We'll walk through a case study and look at why you might want to consider R, how to get started analyzing and visualizing your data, and where to go to learn more.
-
Contains 1 Component(s) Recorded On: 10/07/2020
Natural Language Processing for Text Analytics Pavel Rutherford, Associate Director of Prospect Management and Analytics, Santa Clara University; Adarsh Salapaka, Student Assistant – Prospect Management and Analytics, Santa Clara University The Prospect Management and Analytics team at Santa Clara University is analyzing communications with its constituents to measure what truly matters to them. This is being accomplished by understanding the sentiment from their responses to various marketing campaigns. In leveraging Natural Language Processing (NLP), we have identified a way to infer where a prospect actually resides in the cultivation cycle and how to optimize their overall cultivation. Not All Heroes Wear Capes: Text Mining for Philanthropic Interests with Excel Rachel Veron, Ph.D., Director of Analytics, LSU Foundation An easy first-step into prospecting for a new fundraising project is scouring the information your organization has stored in contact reports. But how can we do this with super-human speed? Enter fundraising analytics and text mining. Join Rachel Veron, Director of Analytics at the LSU Foundation, to learn about a collaborative special project led by Analytics at the LSU Foundation that developed a text mining system.
-
Contains 1 Component(s) Recorded On: 10/07/2020
Join this case study presentation on data analytics driving campaign and organizational strategy. Learn about Marts & Lundy's methodology to determine campaign feasibility, and how Children's National utilized the results to prepare for a 2020 campaign launch. The session will also cover how they pivoted when COVID-19 hit.
-
Contains 1 Component(s) Recorded On: 10/07/2020
Mapping in Microsoft Power BI to Identify Strategic Travel Areas Kendra Yoder, Senior Research Analyst, Office of Prospect Management & Analytics, Penn State University Using data science and technology for better decision-making: a case study in using Microsoft Power BI as a tool for mapping concentrations of our most promising prospects. This session will highlight how Power BI maps enabled us to strategically determine areas of travel for major gift fundraisers to best deploy resources and efficiently raise money Randomistas Deconstructed: Employing Randomized Control Trial Concepts to Build Better Predictive Models James Cheng, Senior Associate Director, Prospect Identification & Analytics, Dana-Farber Cancer Institute & The Jimmy Fund’s Division of Philanthropy This case study details the use of concepts germane to randomized control trial (RCT) set-ups: 1) creating a randomly selected sample from the database equal to the size of the outcome of interest, and 2) controlling for specific characteristics via statistical matching. The case study will compare and evaluate modeling results without and with RCT concept implementation.